What is a NeRF and how can this technology help VR, AR, and the metaverse
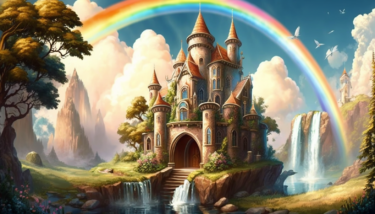
NeRF technology can accelerate the growth of the metaverse, providing more realistic objects and environments for VR and AR devices.
Millions of connected polygons make up the 3D shapes you see in games and virtual worlds such as Horizon Call of the Mountain. Each object is colored, shaded, and textured to create a convincing simulation. While this works, achieving realism is challenging, and some games, like Pistol Whip use stylized graphics instead.
The real world is composed of imperfect, flowing shapes. Even the simple form of a cardboard box rounds at the edges, while a quickly modeled 3D box has rigid, 90-degree angles. The surface could have tiny flaws, crumples, and creases. The box's texture is uneven and fibrous, reflecting light in a diffuse, brown tint that's subtle but visible in certain circumstances.
Natural light reflects and bounces in an incredibly complex orchestra of radiance that is difficult to recreate in a computer simulation. Ray tracing solves this problem by simulating millions of light rays as they ricochet and scatter off objects to create detailed and realistic images. The results are stunning; however, processing a complicated render with real-time ray tracing for a game or VR world requires massive graphics performance.
A new solution has arrived in the form of NeRF technology, a fresh approach to the problem of recreating real-world objects inside a computer.
Content
What is a NeRF?
NeRF is an acronym for Neural Radiance Fields. It's an apt name that includes a large amount of information. Radiance is another word for light, and just as ray tracing recreates the play of light and shadow convincingly, radiance fields achieve the same realism in a new way. A field of radiance represents light from every angle and at every point in a simulation.
That means you can fly a virtual camera through a NeRF and see realistic representations of people, objects, and environments as if you were physically present at that moment in time. As with many computer representations, the quality varies, so NeRFs that are generated quickly or with fewer source images might appear noisy, and the image could have tears. The overall realism of the intact portion of the scene is excellent, however.
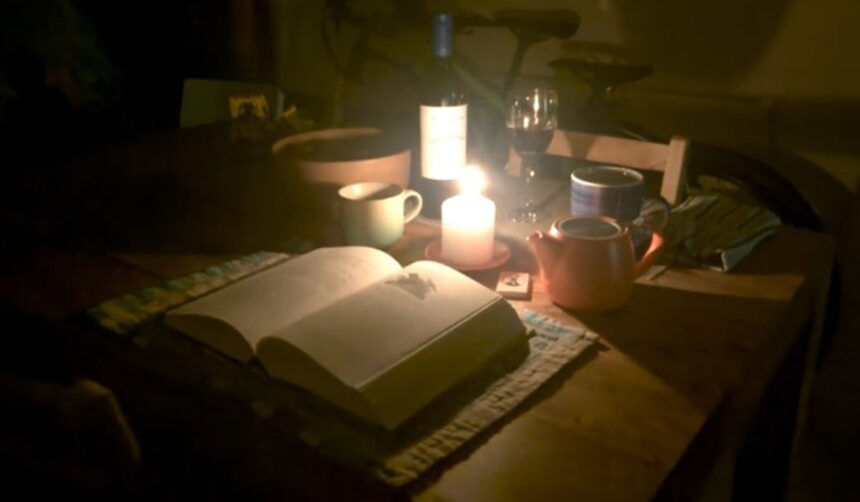
RawNeRF: Google's new image AI brings light to darkness | Image: Ben Mildenhall / Google
Color blotches and blocky artifacts reduced the quality of early JPEG photographs, and the first wave of NeRF renders have similar flaws. As the technology matures, those glitches will become less common.
Another critical detail in the name, NeRF, is the neural rendering required to make this technique possible on low-power devices. While rendering a high-resolution, photorealistic scene at a high frame rate with polygonal ray tracing requires an expensive graphics card, a high-quality NeRF can be rendered on your phone and in a web browser.
That capability to generate realistic 3D imagery quickly, with inexpensive and efficient hardware, makes NeRF technology attractive.
How to capture real-world content for a NeRF
To create a NeRF, you start by taking a series of photographs from various angles throughout an environment or around an object. In some cases, it's more convenient to record a video. This allows you to make NeRFs with video captured from a drone or any other prerecorded content. You can even use this technology to recapture 3D game content as NeRFs.
To achieve the best results, slowly move around any objects of interest, remembering to circle from above, the middle, and below. A NeRF creation app then uses these photos or videos to train an artificial intelligence model to recreate virtual objects inside your computer, phone, or VR headset.
How NeRFs can help build the metaverse
Since this technology is still evolving, viewing NeRFs in VR headsets is in the early stages of development. But the use of NeRFs to build virtual worlds or the metaverse seems almost inevitable.
While some skeptics have trouble visualizing connected virtual worlds, many can appreciate what currently might seem like a futuristic vision of svelte AR glasses enabling virtual objects to appear as detailed, solid, and natural as reality.
Remember the example above and the surprisingly complicated shapes, textures, and lighting effects of a simple object like a cardboard box? Imagine a scene filled with jewelry, chandeliers, stained glass, multiple lights, and mirrors. This added complexity can easily overwhelm a powerful computer with an expensive GPU, causing the frame rate to suffer.
To prepare for a metaverse filled with detailed, realistic objects and rich environments, casting reflections, shadows, and all of the nuances of the natural world, we need a more advanced solution that's less demanding than ray tracing millions of beams of light through scenes filled with millions of polygons.
What about photogrammetry and LiDAR scans?
Photogrammetry and LiDAR scans require a large number of photos or videos recorded in the same circling method used for NeRFs. LiDAR uses lasers to estimate distances for AR, and is used by some photogrammetry apps to speed up processing.
While the capture technique is similar to NeRFs, photogrammetry mathematically aligns images to create a 3D representation of the object known as a point cloud.
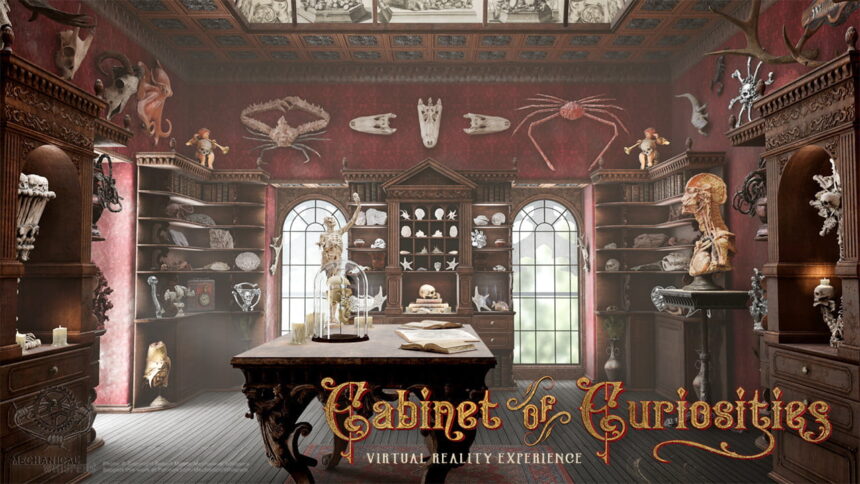
Robert Morris' Virtual Reality Cabinet of Curiosities features hundreds of rare and wondrous objects digitized through photogrammetry. | Image: Mechanical Whispers
Point clouds can capture color and texture accurately, but fail to recreate radiance. When light glints off of a crystal, that happens at a particular angle to the light.
These older technologies are great for the first stage of capture. To generate a photorealistic model, a point cloud is converted to a polygonal model, hand-tuned by a 3D artist, and rendered with ray tracing.
With NeRF technology, all of the nuances of light become part of the capture, and realism is automatic.
Recent advances make NeRFs fast and easy
NeRF technology has been around for several years. The concept of a light field was first described in 1936 by physicist Andrey Gershun. In the last few years, neural processing has exploded as a solution to many computing challenges. AI advances like image and text generation, computer vision, and voice recognition rely upon neural processing to handle the natural world's complex and nearly unpredictable nature.
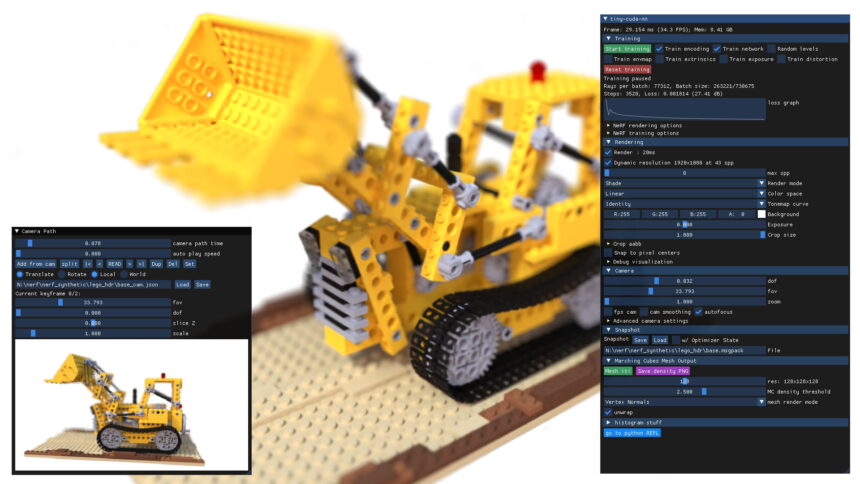
Nvidia's researchers demonstrate a new AI method designed to enable the efficient use of artificial intelligence in computer graphics. | Image: Nvidia
In the early days, neural rendering was time-consuming. Now that computers, mobile, and VR headsets include dedicated neural cores in their central processors and graphics chips, displaying NeRFs has become quick and easy. Nvidia's Instant-NGP provides immediate results, compiling photos and training the NeRF almost instantaneously.
Even an iPhone can capture and create NeRFs with the Luma AI app. Recent advances from Google are making NeRF technology faster, and Meta's NeRF research requires fewer photos to generate a convincing representation.
As NeRF technology continues to advance and become more versatile, neural rendering will likely play a significant role in building the virtual objects and environments that will fill the metaverse and could make VR headsets and AR glasses a necessity in the future.
How can I create NeRFs myself?
Read our guide and learn how to create and see NeRFs in a VR headset.
Note: Links to online stores in articles can be so-called affiliate links. If you buy through this link, MIXED receives a commission from the provider. For you the price does not change.